On July 7th, I defended my Ph.D. thesis in Advanced Computer Technologies

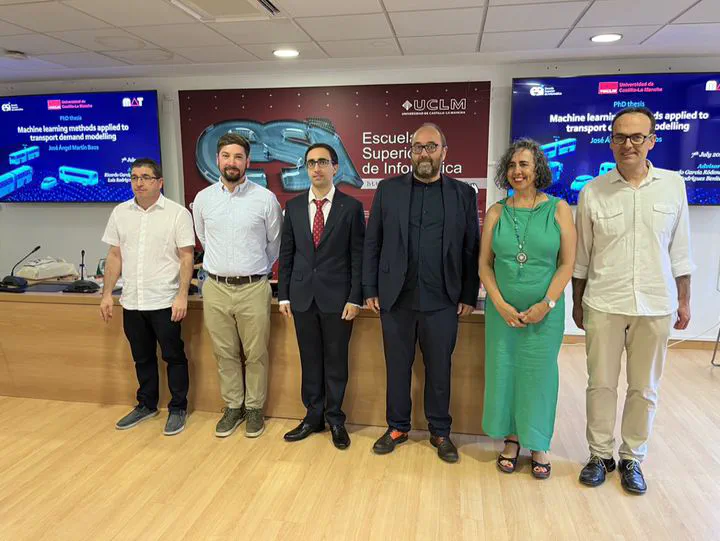
Images
On Friday, July 7, 2023, I successfully defended my doctoral thesis titled ‘Machine learning methods applied to transport demand modelling,’ under the supervision of Professors Ricardo García Ródenas and Luis Rodríguez Benítez. The doctoral thesis, which has received the distinction of international mention, has been evaluated by the committee with the highest grade, receiving a unanimous decision of summa cum laude.
Abstract
Transport demand modelling plays a critical role in transportation planning, enabling the accurate prediction of future transport demand and the evaluation of transport policies and infrastructure plans. However, as transport systems become increasingly complex and recent advances in technology result in massive data collection, traditional analytical methods like Random Utility Models (RUMs) are no longer sufficient to manage this complexity. Therefore, it is necessary to incorporate new techniques to overcome this limitation. This thesis investigates the potential of Machine Learning (ML) methods in this context.
Firstly, it is analysed whether state-of-the-art ML models such as artificial neural networks, support vector machines, and ensemble methods like random forests or gradient boosting decision trees, are superior to RUMs in this research field. To achieve this, the models are compared considering as differential criteria the predictive performance and the ability to derive indicators of decision-makers’ behaviour, always in the context of transport demand modelling.
The results show that classical techniques are outperformed by ML models, but also show that the latter have difficulties in generating reliable econometric indicators. For this reason, a ML model called Kernel Logistic Regression (KLR) is proposed as an alternative to model the utility functions of RUMs, enabling the derivation of econometric indicators. The experiments conducted demonstrate that KLR provides good results on real-world datasets used in previous comparisons in the literature, while providing unbiased estimates of behavioural indicators.
Additionally, it is proposed to extend the application of KLR to a wider range of ML problems by means of an extension of the KLR models called Generalized Kernel Logistic Regression (GKLR). For instance, the GKLR theory has led to the derivation of a novel model called Nested Kernel Logistic Regression (NKLR), which enables the application of KLR to datasets with hierarchically structured data.
Finally, this thesis addresses one of the main limitations of the KLR method, which is the high computational and spatial complexity in large-scale problems. To overcome this limitation, it is suggested the use of the Nyström technique and the implementation of accelerated versions of line search training methods. The results demonstrate that by incorporating these techniques, KLR can efficiently tackle large-scale problems involving hundreds of thousands of data points.