Robustez, eficiencia y recuperación de sistemas de transporte público
Funding Entity | Participants | Duration |
---|---|---|
Ministerio de Ciencia e Innovación. | • Universidad Politécnica de Cataluña. • Universidad de Castilla-La Mancha. • Universidad Rey Juan Carlos. | 30/12/2016 — 29/12/2020 |
The efficiency and reliability of a public transportation system (urban or interurban) depends on the infrastructure design, planning, management and operation and supply of services, all in relation to passenger demand. These features directly affect economic activity, in the use habits of users and has a major impact on environmental issues. Two strategies are essential for improving them: i) increasing the reliability of infrastructure and transport operations and ii) the definition of an offer of transport services aimed at potential demand and framed in the context of competition or cooperation. First strategy i) is conditioned by the levels of fleet maintenance and infrastructure, but both characteristics, robustness and resilience in case of disruptions, as well as strategy ii) are conditioned by design and planning tasks of the transport networks.
This research project is focused on the development of Operations Research techniques which increase the efficiency and reliability of urban and intercity public transport systems in the context of competition or cooperation. Specifically, the levels of strategic and tactical planning under a robust / recoverable point of view are addressed: a) problems of network design and services for metro and rail through stochastic programming with failure scenarios and their associated recovery and models of risk aversion. Urban bus systems are also analyzed but incorporating aspects of congested transit passenger assignment; b) flight programs and rail time-tables considering competition and cooperation in aerial/high speed train modes; c) models for airport and airspace design and management. In the field of operational models, the project develops a) dynamic models for lines of auxiliary services in case of disruptions, b) recovery of delays in air transport and management of operations in the terminal area of airports and c) recovery of incidents for fuel supply problems in the parking areas.
The formulation of previous models leads necessarily to take into account simultaneously with design aspects, tactical and operational questions and to propose formulations integrating both. To this aim various decomposition techniques in mathematical programming (Benders) and distributed surrogate-based optimization are investigated in order to achieve computational viability for solving these problems.
Previous models require a representation of transport demand. This aspect is analyzed in the project under the current approach to big data and machine learning. At operational level, a methodology for estimating dynamically the passengers’ demand will be analyzed by Kalman filtering taking into account the possibilities of tracking end-to-end passenger trips, a possibility that each time appears more clearly offered by using mobile telephony in cooperation with advanced public transport systems and large databases. At tactical and operational level, IVM (importance vector machines) based discrete choice models are investigated for their application to the estimation of demand models for competing modes in a context of intercity transport.
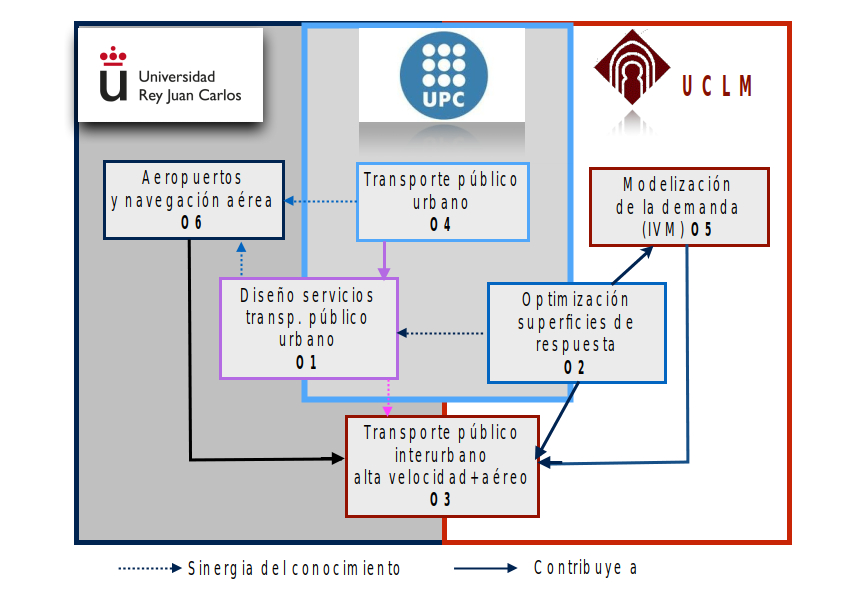
Objective O1 (UPC+URJC): | Objective O2 (UCLM+URJC): | Objective O3 (UCLM+URJC): |
---|---|---|
Design of networks and services with criteria of robustness and recoverability in public transport systems. | Distributed surrogate optimization. | Management of airlines and high-speed trains. Design of flight schedules and service tables with criteria of robustness, recoverability, and risk management in multimodal environments in competition and cooperation. |
Objective O4 (UPC): | Objective O5 (UCLM): | Objective O6 (URJC): |
Operational adaptability of conventional public transport systems to demand variability and estimation of dynamic demand. | Application of machine learning techniques for transport demand modeling in a big data context. | Robust design and incident recovery at airports and in airspace. |