A methodology for monitoring traffic flow and air pollution in urban areas
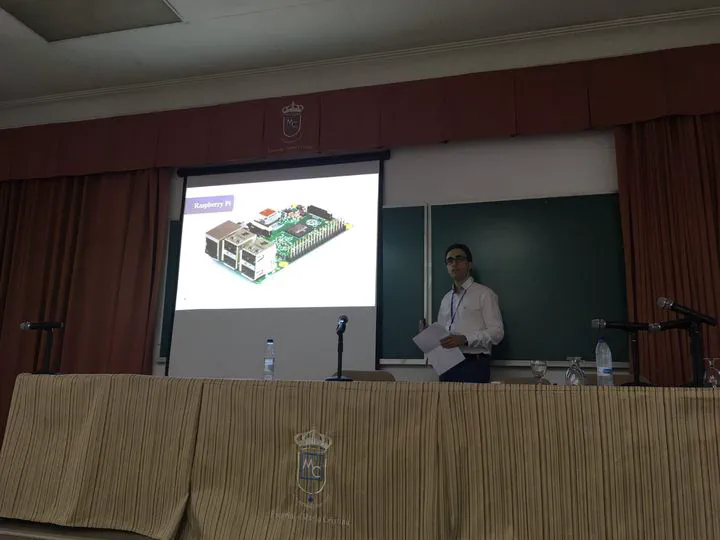
Abstract
Road transportation has become the main source of air pollution in urban areas, which has a major impact on local air quality and human health. For this reason, it is increasingly necessary to accurately estimate the contribution of road transport to air pollution in the cities, so that pollution-reduction measures can be properly designed and implemented appropriately. These pollution reduction measures are becoming increasingly important due to the continued growth in vehicle use and the deterioration of driving conditions (traffic congestion). Authorities find it difficult to meet their environmental objectives and, therefore, reliable mathematical emission models are needed to accurately predict the impact of road transport on air pollution.
Nowadays, intelligent cities are essential to prevent high-level pollution situations and to act when such situations occur. Cities must anticipate pollution peaks and take mitigating measures, such as restricting traffic to a certain number of vehicles or according to their license plates, closing traffic on certain streets, reducing speed limits, etc. In addition, traffic flows must be monitored as they affect pollution levels in that city.
Typical pollution monitoring and control systems often consist of large and expensive devices that are only limited to a few points in the city, hence, they provide information for vast areas and sometimes these systems are not scalable. However, cities are distributed environments where events occur in real time and on a massive scale. Therefore, cities should rely on a low-cost Internet of Things (IoT) infrastructure connected to a cloud platform that supports this type of systems, as well as sensor-based big data applications. These pollution control systems can be combined with a traffic monitoring infrastructure to provide a complete system that can be used as a Decision Support System (DSS) to help authorities make decisions about the environmental impacts caused by pollution before they occur.
In this work, a methodology has been developed for determining the traffic flow and the air pollution in several streets of a city using low-cost devices. An artificial intelligence heuristic algorithm has been employed to process video images from a camera and estimate the traffic flow in the street. This algorithm uses statistical techniques to process the motion vectors generated by the GPU when the video is encoded. Moreover, the computational time required by the algorithm is in the order of 2.5 milliseconds, which allows to count the number of vehicles in real time. This algorithm has been tested proving 90% of accuracy. Finally, this information is uploaded to a cloud service where machine learning techniques can be applied to predict the pollution levels in the city or recommend palliative actions.