Nyström-based approximations for kernel logistic regression: Application to transport choice modelling
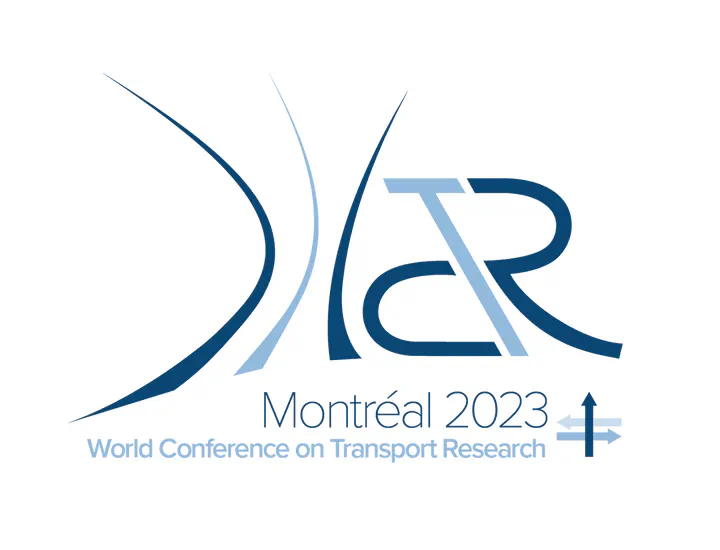
Abstract
The application of machine learning techniques, more specifically kernel-based techniques, to discrete choice modelling using large datasets is limited by the great number of parameters to be considered when building the kernel matrix and the size of the kernel matrix itself. The spatial and temporal complexity is such that these methods are not applicable to large sample sizes. However, there are techniques that allow generating a low-rank matrix approximation to the kernel matrix, one of them is the Nyström method. One limitation of the Nyström method is that the quality of the kernel matrix approximation depends on the proper choice of landmark points. In this work, four variants of this technique are implemented, a basic uniform method, one based on the K-means algorithm and two different implementations of a non-uniform method based on leverage scores. Later, in the experimentation, we conduct a comparison of these methods applied to two big transport mode choice datasets, which contain a large number of samples and variables. Finally, these results are compared with Multinomial Logit and other techniques currently relevant in the Machine Learning field.